Personalization and Recommendations in 'Where To Buy' Solutions
Table of Contents
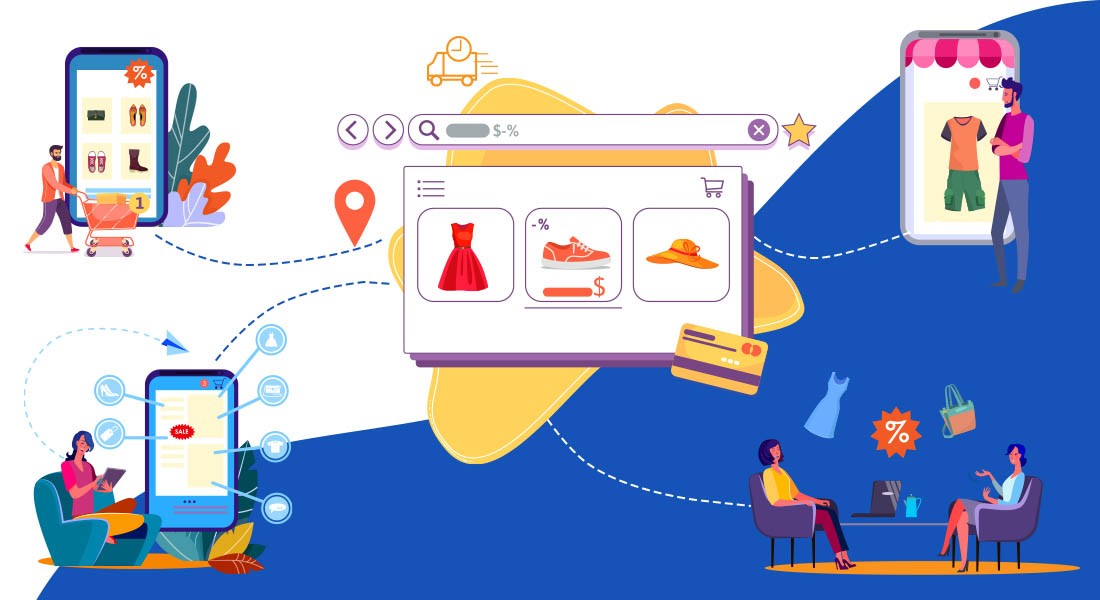
Introduction
In the ever-evolving realm of e-commerce, providing a personalized and relevant shopping experience has become paramount. This phenomenon has been powered by the integration of advanced technologies and smart algorithms into ‘Where To Buy’ solutions. In this blog post, we embark on a journey to explore the pivotal role of personalization and recommendations in ‘Where To Buy’ solutions and how they are reshaping the way consumers shop online.
E-commerce has witnessed a meteoric rise, and with the convenience of online shopping comes an abundance of choices for consumers. Navigating this digital marketplace can be overwhelming, often leading to decision fatigue and cart abandonment. To address these challenges and provide a tailored shopping experience, businesses have turned to personalization and recommendation systems.
The foundation of personalization lies in understanding and anticipating the unique preferences, behaviors, and needs of each individual shopper. This goes beyond merely addressing customers by their first name in marketing emails. It’s about leveraging data and technology to curate product recommendations, content, and interactions that resonate with each shopper on a personal level.
Recommendation systems, on the other hand, are the digital wizards behind the scenes, tirelessly analyzing vast amounts of data to suggest products and content that align with a customer’s interests. These systems take into account factors like past purchases, browsing history, demographic information, and even real-time behavior to provide tailored suggestions.
The benefits of personalization and recommendations extend to both sides of the e-commerce transaction. For consumers, it means a more enjoyable and efficient shopping journey. They are more likely to discover products they love, save time, and feel understood and valued by the brand. For businesses, it translates into increased sales, higher customer retention, and a deeper understanding of customer behavior.
In this blog post, we will delve deeper into these aspects. We’ll explore the technology and algorithms that power personalization and recommendations. We’ll also look at real-life examples of businesses that have harnessed these tools effectively, shedding light on the tangible benefits they’ve reaped. Additionally, we’ll consider the challenges, such as data privacy concerns, that businesses must navigate when implementing these features.
As we navigate through the landscape of personalization and recommendations in ‘Where To Buy’ solutions, it’s essential to recognize that we’re not just talking about features or algorithms. We’re exploring how technology is making the online shopping experience more human, more intuitive, and ultimately, more satisfying. So, let’s embark on this journey to uncover the magic that happens behind the ‘Where To Buy’ buttons.
The Role of Personalization
Personalization has become the heartbeat of modern e-commerce, revolutionizing the way online shoppers interact with products and brands. In this chapter, we’ll dive deep into the pivotal role that personalization plays within ‘Where To Buy’ solutions and how it transforms the shopping experience.
Understanding the Shopper
At the core of personalization is the ability to understand each shopper on a profound level. It involves collecting and analyzing data points such as:
- Browsing History: Tracking the products a shopper has viewed provides insights into their interests.
- Purchase History: Understanding what customers have bought in the past helps predict their future preferences.
- Demographic Information: Age, gender, location, and other demographic data help tailor recommendations.
- Behavioral Data: Real-time behavior on the website, such as clicks, time spent on pages, and interaction with specific products, provides immediate insights.
Product Recommendations
One of the most visible aspects of personalization is product recommendations. Based on the data collected, sophisticated algorithms can suggest products that align with a shopper’s preferences. These recommendations can manifest in various ways:
- Product Pages: Suggesting related or complementary products on the product detail page.
- Shopping Carts: Encouraging upsells or cross-sells when a product is added to the cart.
- Homepage: Featuring personalized product carousels or sections showcasing items of interest.
- Email Marketing: Sending personalized product recommendations via email, reminding customers of their interests.
Content Personalization
Beyond products, content personalization is equally crucial. Tailoring the content a shopper encounters, such as blog articles, videos, or guides, enhances their engagement. For instance, if a customer frequently reads about fitness, a personalized homepage might showcase fitness-related articles or workout equipment.
Personalized Pricing and Promotions
Personalization extends to pricing and promotions. ‘Where To Buy’ solutions can dynamically adjust prices based on user behavior and history. For example, a first-time visitor might see a different price than a loyal customer. Tailored promotions, like exclusive discounts on frequently purchased items, can also be employed to incentivize sales.
Enhancing User Experience
Personalization isn’t limited to recommendations and content. It extends to the entire user experience. This includes:
- User Interface: Adapting the layout, color schemes, and design elements based on user preferences.
- Search Results: Prioritizing products in search results that align with a user’s history and preferences.
- Navigation: Simplifying or customizing website navigation based on user behavior.
The overarching goal is to make the shopping experience as effortless and enjoyable as possible.
Building Customer Loyalty
A well-executed personalization strategy fosters customer loyalty. When shoppers feel understood and catered to, they are more likely to return. This loyalty results in higher customer lifetime value, as repeat customers tend to spend more over time.
In this era of data-driven decision-making, personalization is the secret sauce that empowers ‘Where To Buy’ solutions. It transforms online shopping from a generic, one-size-fits-all experience into a personalized journey that leaves shoppers satisfied and coming back for more. The next chapter will delve into the technology that fuels this personalization magic.
Understanding Recommendation Systems
In the realm of ‘Where To Buy’ solutions, the engine driving personalization and product suggestions is the recommendation system. These systems are the digital brains behind the scenes, working tirelessly to analyze data and serve up personalized product recommendations. In this chapter, we’ll explore recommendation systems in depth, uncovering how they function and why they’re essential for enhancing the shopping experience.
How Recommendation Systems Work
At their core, recommendation systems are algorithms designed to predict a user’s preferences or needs and suggest items that align with those predictions. The fundamental premise is relatively simple: if a system can understand a user’s preferences, it can recommend products that cater to those preferences.
There are primarily two types of recommendation systems:
Collaborative Filtering: This method relies on user behavior and historical data. It identifies users who have similar preferences and suggests products based on what similar users have liked or interacted with. Collaborative filtering can be further divided into user-based and item-based approaches.
Content-Based Filtering: In this approach, the system takes into account the attributes of products and the user’s past behavior. If a user has previously shown interest in a specific category or brand, the content-based recommendation system will suggest items with similar attributes.
The Importance of Data
The lifeblood of recommendation systems is data. They require a robust dataset that includes user profiles, behavior, and product attributes. Here’s the kind of data that fuels these systems:
User Data: This includes demographic information, browsing history, purchase history, and user-generated content like reviews and ratings.
Product Data: Details about products, such as category, brand, specifications, and any available metadata.
Interaction Data: Information about how users interact with products, such as clicks, views, and purchases.
The Role of Machine Learning
Machine learning algorithms are often at the heart of recommendation systems. These algorithms analyze vast amounts of data to identify patterns and make predictions. The more data the system has, the better it becomes at making accurate recommendations.
Personalization at Scale
What makes recommendation systems so powerful is their ability to personalize at scale. Even on an e-commerce site with millions of products and a large user base, these systems can provide tailored recommendations to each individual.
Benefits of Recommendation Systems
Increased Sales: By suggesting products that align with a user’s interests, recommendation systems boost conversion rates and drive more sales.
Enhanced User Experience: Shoppers appreciate the convenience of finding relevant products quickly, improving their overall experience.
Customer Retention: Personalized recommendations can keep customers engaged and coming back for more, increasing customer loyalty.
Data-Driven Insights: These systems generate valuable insights into user behavior, helping businesses make informed decisions about inventory, marketing, and product development.
In summary, recommendation systems are the secret sauce that powers the personalization engine within ‘Where To Buy’ solutions. They transform the online shopping experience by helping users discover products they might not have found otherwise, increasing sales, and building brand loyalty. The next chapter will explore the challenges and considerations associated with implementing recommendation systems effectively.
Benefits of Personalization and Recommendations
Personalization and recommendation systems in ‘Where To Buy’ solutions offer a plethora of advantages for both businesses and consumers. In this chapter, we’ll delve into the numerous benefits that arise from implementing these powerful tools.
1. Enhanced Customer Experience:
One of the most immediate and evident benefits of personalization and recommendation systems is the significantly improved customer experience. By tailoring product recommendations to individual preferences, users feel more valued and understood. This customization adds a personal touch to the shopping journey, making it more enjoyable and relevant.
2. Increased Sales and Revenue:
Perhaps the most critical advantage for businesses is the boost in sales and revenue. Personalized product recommendations have been shown to increase conversion rates and average order values. When customers see products that align with their interests or needs, they are more likely to make a purchase. This translates to higher revenue streams and a healthier bottom line.
3. Improved Customer Retention:
Personalization plays a pivotal role in building customer loyalty. When users consistently receive relevant recommendations, they are more likely to return to the platform or e-commerce site. This not only boosts retention rates but also reduces customer churn, a critical metric for long-term success.
4. Efficient Inventory Management:
For businesses, personalization systems provide insights into product popularity and customer preferences. This data can be leveraged to optimize inventory management. Companies can focus on stocking products that have a higher likelihood of being purchased, reducing overstocking and waste.
5. Data-Driven Decision-Making:
Personalization and recommendation systems generate vast amounts of user data. This data can be mined for valuable insights into customer behavior, preferences, and trends. Businesses can use these insights to make data-driven decisions regarding marketing strategies, product development, and customer targeting.
6. Cross-Selling and Upselling:
These systems are adept at suggesting complementary products or upgrades, leading to cross-selling and upselling opportunities. For example, if a customer adds a camera to their cart, the system might recommend additional lenses, memory cards, or a camera bag. This increases the average transaction value.
7. Time and Effort Savings:
Customers appreciate the convenience of finding what they need quickly. Recommendation systems save users time and effort by presenting relevant products without the need for extensive searches. This streamlined experience can be a significant selling point for your platform or e-commerce site.
8. Competitive Edge:
In today’s highly competitive market, businesses that offer personalized shopping experiences gain a significant competitive edge. Customers are more likely to choose platforms that understand their preferences and provide tailored recommendations over generic alternatives.
9. Adaptable to Customer Lifecycle:
Personalization and recommendation systems can adapt to different stages of the customer lifecycle. They can provide product suggestions for first-time visitors, returning customers, and even re-engage inactive users, ensuring that recommendations remain relevant over time.
10. Increased Customer Engagement:
When users receive recommendations that align with their interests, they are more likely to engage with the platform, browse more products, and spend more time exploring. This heightened engagement can lead to a stronger brand-consumer relationship.
In conclusion, the benefits of personalization and recommendation systems in ‘Where To Buy’ solutions are extensive. They encompass improved user experiences, increased sales and revenue, better customer retention, and data-driven decision-making. These systems are a win-win for both businesses and consumers, creating a more tailored, efficient, and satisfying shopping journey. In the next chapter, we will discuss the challenges and considerations involved in implementing personalization and recommendation systems effectively.
Tailoring Product Suggestions
In the world of ‘Where To Buy’ solutions, tailoring product suggestions is a critical component of personalization and recommendation systems. This chapter explores the intricacies of how product recommendations are customized to meet the unique preferences and needs of individual users.
1. User Behavior Analysis:
The foundation of tailoring product suggestions lies in user behavior analysis. This involves tracking and understanding how users interact with a platform or e-commerce website. By monitoring actions such as product views, searches, clicks, and purchases, recommendation systems can gather valuable data on individual preferences.
2. Collaborative Filtering:
Collaborative filtering is a common technique used in tailoring product suggestions. It leverages historical user data to identify patterns and relationships between users and products. If User A and User B have similar preferences and have both shown interest in Product X, the system may recommend Product X to User A based on User B’s past behavior.
3. Content-Based Filtering:
Content-based filtering, on the other hand, focuses on the attributes of products and the user’s profile. It recommends products similar to those the user has previously interacted with. For example, if a user frequently views and buys running shoes, the system may suggest other athletic footwear options.
4. Hybrid Approaches:
Many recommendation systems use hybrid approaches that combine collaborative and content-based filtering. This offers a more comprehensive and accurate understanding of user preferences. By considering both user behavior and product attributes, these systems provide more relevant and diverse recommendations.
5. Real-Time Personalization:
Tailoring product suggestions can also involve real-time personalization. This means that recommendations are continuously updated as users interact with the platform. For example, if a user is currently searching for hiking boots, the system will prioritize suggesting hiking-related products over unrelated items.
6. Seasonal and Trend-Based Recommendations:
Recommendation systems can take into account seasonal trends and user-specific preferences for different times of the year. During the holiday season, for instance, the system may emphasize gift ideas and festive products. This adaptability keeps recommendations relevant and engaging.
7. Cart and Purchase History:
The user’s cart and purchase history are valuable sources of information for tailoring product suggestions. If a customer has added items to their cart or purchased products in the past, the system can recommend complementary or related items. For instance, if a user buys a camera, the system may suggest lenses or camera bags.
8. User Feedback Integration:
Some recommendation systems incorporate user feedback and ratings into their algorithms. If a user rates products or provides feedback, the system can use this information to refine future recommendations. Positive feedback on certain items can lead to more frequent recommendations of similar products.
9. Diverse Recommendations:
While tailoring product suggestions is about personalization, it’s essential to strike a balance. Overly personalized recommendations can become repetitive and limit users’ exploration. Therefore, recommendation systems aim to offer a mix of familiar and serendipitous product suggestions to keep the shopping experience fresh and exciting.
10. Privacy and Transparency:
As personalization becomes more sophisticated, addressing user concerns about privacy and transparency is crucial. Users should have control over the level of personalization and be aware of how their data is used to tailor recommendations.
In summary, tailoring product suggestions in ‘Where To Buy’ solutions involves a deep understanding of user behavior, collaborative and content-based filtering techniques, real-time personalization, and the integration of user feedback. It’s a delicate balance between delivering relevant recommendations and respecting user privacy and choice. In the next chapter, we will explore the challenges and considerations associated with implementing personalized product suggestions effectively.
Enhancing User Experience
In the realm of ‘Where To Buy’ solutions, enhancing user experience is paramount to success. User experience (UX) encompasses every aspect of a user’s interaction with a digital platform, from the initial visit to the final purchase. This chapter delves into the strategies and practices used to create a seamless and enjoyable shopping journey for users.
1. Intuitive User Interface (UI):
A well-designed and intuitive user interface is the cornerstone of an excellent user experience. Users should be able to navigate the platform effortlessly, find products easily, and understand how to make purchases. Clear and concise menus, search bars, and product categorization all contribute to a user-friendly UI.
2. Fast Loading Speeds:
Slow-loading pages can be a significant turnoff for users. Ensuring that the platform loads quickly, especially on mobile devices, is crucial. This includes optimizing images, minimizing server response times, and utilizing content delivery networks (CDNs) to distribute content efficiently.
3. Mobile Responsiveness:
In today’s mobile-centric world, a mobile-responsive design is imperative. ‘Where To Buy’ solutions must adapt seamlessly to various screen sizes and orientations, providing a consistent and enjoyable experience on smartphones and tablets.
4. Clear Product Information:
Detailed and transparent product information is vital for users making informed purchase decisions. This includes high-quality images, comprehensive product descriptions, pricing, availability, and customer reviews. User-generated content, such as reviews and ratings, adds credibility.
5. Efficient Search Functionality:
The search functionality should be efficient and accurate. Users often rely on search bars to find specific products quickly. Implementing auto-suggestions, filters, and advanced search capabilities can significantly improve the search experience.
6. Personalization and Recommendations:
As discussed in previous chapters, personalization and recommendations enhance user experience by presenting relevant product suggestions. When users feel that the platform understands their preferences, they are more likely to engage and convert.
7. Simplified Checkout Process:
A lengthy or complicated checkout process can lead to cart abandonment. Streamlining the checkout process, offering guest checkouts, and providing multiple payment options can reduce friction and improve the overall experience.
8. Security and Trust:
Users need to trust the platform with their personal and financial information. Implementing robust security measures, such as SSL certificates and secure payment gateways, is essential. Displaying trust signals like security badges and privacy policies can also boost confidence.
9. Customer Support and Assistance:
Responsive and accessible customer support channels are critical. Users may have questions or encounter issues during their shopping journey. Providing live chat, email support, or a comprehensive FAQ section can address user needs promptly.
10. Feedback and Continuous Improvement:
Finally, listening to user feedback and continuously improving the platform is an ongoing process. Conducting user surveys, monitoring user behavior, and analyzing data can reveal pain points and areas for enhancement. A commitment to refinement is a hallmark of platforms that prioritize user experience.
In conclusion, enhancing user experience in ‘Where To Buy’ solutions involves creating an intuitive interface, optimizing performance, providing comprehensive product information, and implementing personalization, among other strategies. A positive user experience not only drives conversions but also fosters customer loyalty and advocacy. In the next chapter, we will explore the impact of mobile shopping and how to optimize for this growing segment of users.
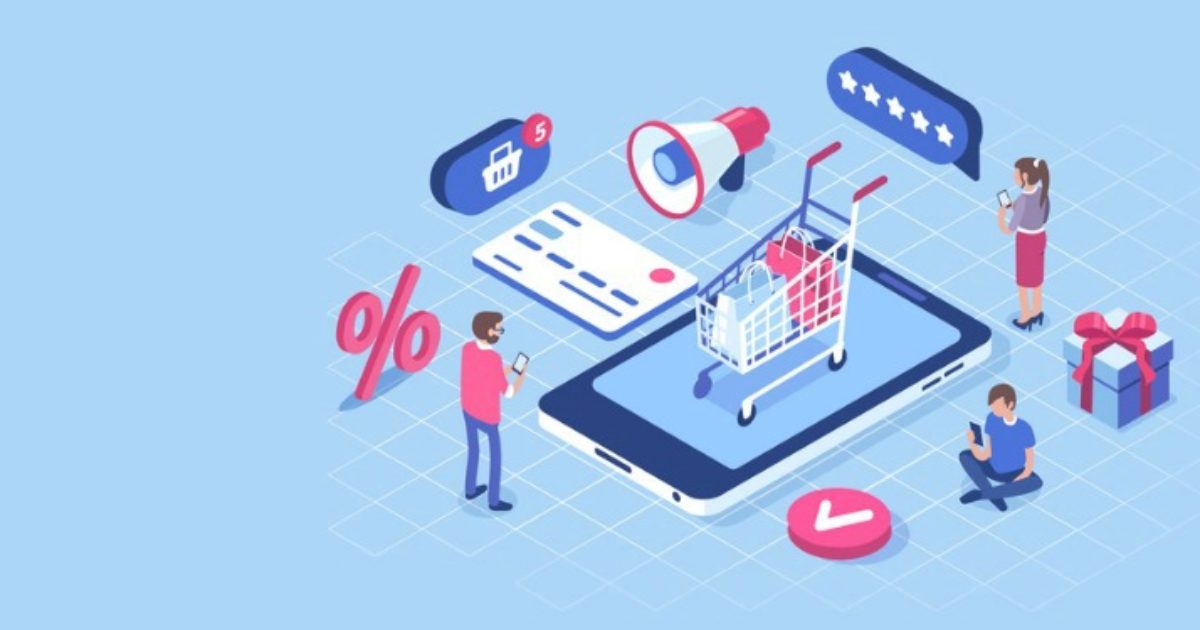
The Technology Behind Personalization
The success of personalization and recommendation systems in ‘Where To Buy’ solutions relies heavily on advanced technology and algorithms. In this chapter, we delve into the technology that powers the personalization of user experiences.
1. Machine Learning and Artificial Intelligence (AI):
Machine learning and AI are at the core of personalization technology. These systems analyze vast amounts of data, including user behavior, preferences, and historical interactions, to make predictions and recommendations. Through techniques like natural language processing (NLP) and deep learning, these algorithms become more accurate over time.
2. Data Collection and Processing:
To offer relevant recommendations, personalization systems collect and process a multitude of data points. These include user demographics, browsing history, search queries, and purchase history. This data is then structured and analyzed to identify patterns and preferences.
3. Collaborative Filtering:
Collaborative filtering is a popular recommendation technique that identifies users with similar preferences. When a user interacts with products or content, the system identifies other users who have shown similar behavior and recommends items they have liked. This method is particularly effective for discovering new products.
4. Content-Based Filtering:
Content-based filtering focuses on the attributes of products and users. For example, if a user has shown a preference for a specific brand, style, or category, the system will recommend items with similar attributes. This approach is excellent for personalizing recommendations based on product features.
5. Reinforcement Learning:
Reinforcement learning is a more advanced technique where the system learns from user interactions and feedback. It continually refines recommendations by considering the user’s explicit and implicit preferences. This approach is adaptive and can optimize recommendations over time.
6. Real-Time Data Processing:
Personalization systems often operate in real-time. They process user actions and preferences as they occur, ensuring that recommendations are up to date. Real-time processing requires robust infrastructure and low-latency data handling.
7. Scalable Infrastructure:
As ‘Where To Buy’ solutions cater to a large user base, the technology behind personalization must be highly scalable. Cloud-based solutions and distributed computing enable systems to handle increasing loads efficiently.
8. A/B Testing and Experimentation:
To fine-tune personalization algorithms, A/B testing and experimentation are essential. These methodologies allow companies to test different recommendation strategies and measure their impact on user engagement and conversions.
9. Privacy and Data Security:
With the growing concern over data privacy, personalization technology must adhere to strict data protection regulations. Anonymizing and securing user data is crucial to maintain trust.
10. Feedback Loops:
Feedback loops enable personalization systems to learn from user reactions to recommendations. User feedback, such as liking or disliking a recommendation, helps the system continuously improve its suggestions.
In summary, personalization technology leverages machine learning, data processing, and various recommendation techniques to provide users with tailored experiences. It’s a dynamic field that continually evolves to meet user expectations and adapt to changing preferences. In the next chapter, we will explore the impact of personalization on user engagement and conversion rates in ‘Where To Buy’ solutions.
Challenges and Considerations
While personalization and recommendation systems in ‘Where To Buy’ solutions offer numerous benefits, they also come with their fair share of challenges and considerations. In this chapter, we explore some of the key challenges and important considerations when implementing personalization technology.
1. Data Privacy and Security:
One of the most significant concerns surrounding personalization is the collection and use of user data. Ensuring that user data is handled securely and in compliance with data protection regulations is paramount. Companies must be transparent about data practices and give users the option to control their data.
2. Algorithm Bias:
Personalization algorithms are only as good as the data they are trained on. If the data used to train these algorithms contains biases, it can result in recommendations that reinforce stereotypes or exclude certain groups of users. Addressing algorithm bias is an ongoing challenge.
3. Striking the Right Balance:
Finding the right balance between personalization and user privacy can be challenging. Over-personalization can make users feel uncomfortable, while under-personalization may result in less effective recommendations. Striking the right balance is crucial.
4. Algorithm Transparency:
Users often appreciate understanding why they receive specific recommendations. Ensuring that recommendation algorithms are transparent and explainable can help build trust with users.
5. Scalability:
As user bases grow, personalization systems must scale to accommodate increased data processing requirements. Scalability challenges can arise when handling large volumes of user data in real-time.
6. Diversity of Recommendations:
Avoiding recommendation “bubbles” where users are only exposed to content or products similar to their previous interactions can be a challenge. Systems should strive to provide diverse recommendations to ensure users discover new items.
7. User Engagement:
Not all users will interact with recommendations in the same way. Some may embrace personalized content, while others may ignore it. Keeping users engaged with recommendations is an ongoing effort.
8. Ethical Considerations:
Companies must consider the ethical implications of their personalization strategies. Ensuring that personalization benefits users without exploiting their vulnerabilities or manipulating their behavior is essential.
9. Resource Allocation:
Implementing and maintaining personalization technology can require significant resources, including skilled personnel, infrastructure, and ongoing monitoring. Companies need to allocate resources effectively to maximize the benefits.
10. Continuous Improvement:
Personalization is not a one-time effort but an ongoing process. Systems should be continually updated and improved based on user feedback and changing trends.
In conclusion, personalization and recommendation systems offer tremendous potential for enhancing user experiences in ‘Where To Buy’ solutions. However, addressing the associated challenges and considerations is crucial to ensure that personalization efforts are both effective and ethical. In the next chapter, we will explore real-world case studies of successful personalization implementations in ‘Where To Buy’ solutions.
Success Stories
In this chapter, we’ll delve into real-world success stories of companies that have implemented personalization and recommendation systems in their ‘Where To Buy’ solutions. These stories provide valuable insights into how personalization can drive positive outcomes and benefit both businesses and consumers.
1. Amazon:
Amazon, one of the pioneers in personalization technology, has set a high standard for delivering tailored product recommendations. They leverage machine learning algorithms to analyze user behavior, purchase history, and product interactions. This data powers the “Customers Who Bought This Also Bought” and “Recommended for You” sections. As a result, Amazon sees a significant increase in sales and customer engagement.
2. Netflix:
Netflix relies heavily on personalization to enhance user experience. Its recommendation system analyzes user viewing habits and preferences to suggest personalized movie and TV show recommendations. This approach has not only led to increased user satisfaction but has also played a pivotal role in retaining subscribers.
3. Spotify:
Spotify uses personalization to curate playlists and recommend music to its users. The “Discover Weekly” playlist, generated using machine learning algorithms, provides users with a fresh list of songs every week tailored to their music preferences. This feature has been a massive hit, with millions of users discovering new music regularly.
4. eBay:
eBay’s personalization efforts extend to its homepage and search results. By analyzing user behavior and purchase history, eBay customizes product listings to match each user’s interests. This approach has led to increased click-through rates and higher sales for eBay sellers.
5. Sephora:
Sephora, a beauty retailer, employs personalization to offer tailored product recommendations and beauty tips to its customers. By analyzing skincare and makeup preferences, Sephora provides a highly personalized shopping experience. This approach has driven increased customer loyalty and higher average order values.
6. Stitch Fix:
Stitch Fix, an online clothing retailer, combines personalization with expert styling. Customers fill out a detailed style profile, and Stitch Fix’s algorithmic stylists use this information to select clothing items tailored to each individual’s taste. This unique blend of personalization and expert curation has led to impressive customer satisfaction and retention rates.
7. Airbnb:
Airbnb uses personalization to improve the guest experience. By analyzing previous bookings, preferences, and reviews, Airbnb suggests accommodations that align with a guest’s travel style. These tailored recommendations have resulted in higher booking rates and guest satisfaction.
8. YouTube:
YouTube’s recommendation system uses machine learning to suggest videos that users are likely to enjoy based on their viewing history. This approach keeps users engaged, leading to longer viewing sessions and increased ad revenue.
9. Zillow:
Zillow, a real estate platform, offers personalized property recommendations to users based on their search history and preferences. This personalization has led to higher user engagement and more successful property listings.
10. Walmart:
Walmart employs personalization to tailor its online shopping experience. Recommendations for products similar to those in a user’s cart or based on their browsing history have contributed to increased conversions and higher sales.
These success stories highlight the immense potential of personalization and recommendation systems in ‘Where To Buy’ solutions. By delivering relevant and engaging experiences to users, companies can build stronger customer relationships, boost sales, and stay competitive in today’s dynamic e-commerce landscape. In the final chapter, we’ll wrap up our exploration of personalization and recommendations in ‘Where To Buy’ solutions and provide key takeaways for businesses looking to implement these strategies.
Future Trends
As technology continues to evolve, the landscape of ‘Where To Buy’ solutions is poised for significant changes. In this chapter, we’ll explore some of the future trends and developments that are likely to shape the field of personalization and recommendations in ‘Where To Buy’ solutions.
1. Artificial Intelligence (AI) Advancements:
AI and machine learning will play an even more prominent role in personalization. Advanced algorithms will better understand user behavior, preferences, and context, leading to more accurate product recommendations. AI-powered chatbots and virtual shopping assistants will provide personalized guidance to shoppers.
2. Voice Commerce:
Voice-activated devices like smart speakers are becoming increasingly popular. ‘Where To Buy’ solutions will need to adapt to this trend by enabling voice-activated searches and purchases. Personalized voice recommendations will be a key focus for businesses.
3. Augmented Reality (AR) Shopping:
AR will revolutionize online shopping by allowing customers to virtually try on products before buying. Personalized AR experiences will suggest products that match a user’s style and preferences, enhancing the online shopping experience.
4. Hyper-Personalization:
Personalization will become hyper-specific, with recommendations not just based on past behavior but also on real-time context. For example, a ‘Where To Buy’ solution might consider a user’s location, weather, and current activities to make highly relevant suggestions.
5. Enhanced Data Privacy:
With growing concerns about data privacy, businesses will need to prioritize transparent and secure handling of user data. Future personalization efforts will focus on providing value while respecting user privacy preferences and regulations like GDPR.
6. Social Commerce Integration:
Social media platforms will increasingly integrate with ‘Where To Buy’ solutions. Users will be able to make purchases directly from social posts and stories. Personalized shopping experiences will be based on a user’s social interactions and preferences.
7. Sustainability and Ethical Shopping:
Consumers are becoming more conscious of sustainability and ethics. Future ‘Where To Buy’ solutions will incorporate personalization to highlight eco-friendly and ethically sourced products, aligning with consumer values.
8. Cross-Channel Consistency:
Users often switch between devices and channels while shopping. Personalization will ensure a consistent experience across platforms, making it easy for users to transition from mobile to desktop or from online to in-store.
9. Predictive Analytics:
Advanced predictive analytics will enable businesses to anticipate user needs and desires. By analyzing historical data and external factors, ‘Where To Buy’ solutions will offer products even before users actively search for them.
10. Global Expansion:
Personalization will be crucial for ‘Where To Buy’ solutions expanding into global markets. Understanding cultural nuances and regional preferences will be essential to providing relevant recommendations worldwide.
In conclusion, the future of ‘Where To Buy’ solutions lies in delivering highly personalized and anticipatory shopping experiences. By embracing emerging technologies and trends, businesses can stay competitive and continue to meet the evolving expectations of modern consumers. As we’ve explored throughout this guide, personalization and recommendations are not just trends but essential strategies for success in the world of e-commerce.
FAQs
1. What is the significance of personalization in ‘Where To Buy’ Solutions?
Personalization in ‘Where To Buy’ Solutions is essential because it enhances the user experience by providing tailored product recommendations based on individual preferences. It leads to higher customer satisfaction and increased sales.
2. How do recommendation systems work in ‘Where To Buy’ Solutions?
Recommendation systems in ‘Where To Buy’ Solutions use algorithms to analyze user behavior and preferences. They then suggest products that are likely to be of interest to the user, based on this analysis.
3. Are there any privacy concerns associated with personalization in e-commerce?
Yes, data privacy is a concern. Businesses must handle user data responsibly and transparently. Users should have control over how their data is used, and businesses should comply with privacy regulations like GDPR.
4. What are some challenges in implementing personalization and recommendation systems?
Challenges include collecting and analyzing user data, avoiding filter bubbles, and striking the right balance between personalization and privacy. Businesses also need to keep up with evolving technology.
5. How can businesses stay ahead by embracing future trends in personalization?
To stay ahead, businesses should invest in AI and machine learning, adapt to voice commerce, and explore augmented reality shopping experiences. They should also prioritize data privacy, sustainability, and global expansion in their strategies.